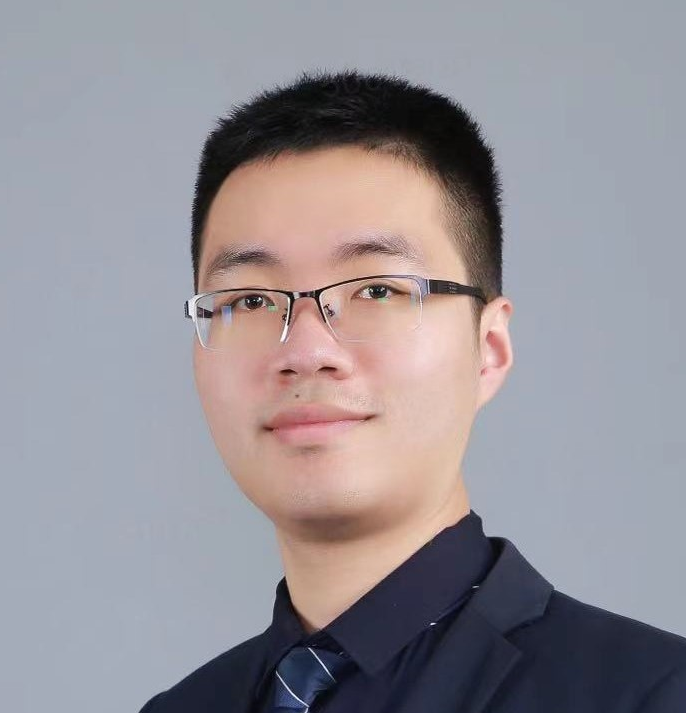
Haizhou Liu , Ph.D.
Southeast University
Lecturer
Contact
Links
CV Download
[PDF-CN]     [PDF-EN]
Education
Year | Degree | Institution | Major | Supervisor | GPA |
2022.09 — 2024.06 | Ph.D. | Tsinghua University | Electrical Engineering | Xuan Zhang | 3.9/4.0 |
2019.09 —2022.06 | Master (Transferred to Ph.D.) | Tsinghua University | Environ Science and New Energy Tech | Hongbin Sun/Xinwei Shen | 3.9/4.0 |
2015.09 — 2019.06 | B.Sc. | Nanjing University | Physics | Lin Zhou/Jia Zhu | 4.7/5.0 |
Overseas Experience
Year | Identity | Institution | Major | Supervisor | GPA |
2023.05 — 2024.01 | Visiting Scholar | UC Berkeley | Computer Science | Somayeh Sojoudi | —— |
2019.09 — 2021.08 | Online Program | U-M Ann Arbor | Applied Data Science | —— | 3.9/4.0 |
2017.08 — 2017.12 | Exchange Student | Duke University | Physics | Sara Haravifard | 3.9/4.0 |
Awards
-
Awarded during Ph.D. study at Tsinghua University:
- 2022.12   Outstanding Graduating Student at Tsinghua University.
- 2022.12   National Scholarship for Graduates.
- 2021.12   Comprehensive Scholarship Award at Tsinghua University, First Prize.
-
Awarded during undergraduate study at Nanjing University:
- 2019.09   Outstanding Graduation Thesis at Nanjing University.
- 2019.05   Outstanding Graduating Student at Nanjing University.
- 2016.11   National Scholarship for Undergraduates.
-
Awarded during internship at Huawei Technologies:
- 2021.06   Huawei Creativitiy Pioneer 2021 (First intern in lab to be awarded this prize).
Research Directions
Smart Grid; Smart Cities; Distributed Machine Learning (Federated Learning); Integrated Energy System Optimization; Privacy Preservation.
Research Experience
-
1. Federated Learning and Its Application in the Smart Grid News
Supervisor: Prof. Xuan Zhang
- Demonstrated the applicability and potentials of federated learning in Smart City load prediction problems.
- Proposed a hybrid federated learning framework based on XGBoost, in order to incentivize homogeneous and heterogeneous data holders to simultaneously join in the collaborative training.
- Designed a multi-task federated learning framework for district load forecasting, with dynamic and simultaneous district dropout mechanisms, respectively.
-
2. Model/Data-Driven Integrated Energy Systems News
Supervisor: Prof. Hongbin Sun/Prof. Xinwei Shen
- Improved the heuristic Progressive Hedging algorithm, in order to accelerate convergence in stochastic electricity-gas coupled scheduling problems.
- Applied artificial neural networks to achieve fast and accurate economic dispatch in an electricity-gas coupled system.
- Proposed a data-driven warm start algorithm for optimal economic dispatch in integrated energy systems.
-
3. Solar-Thermal Conversion based on Nanomaterials News
Supervisors: Prof. Lin Zhou/Prof. Jia Zhu
- Designed a highly efficient solar thermal photovoltaic absorber based on the Optical TAMM State.
- Conducted studies and reviews on nano-scale solar water evaporation.
Internships
-
1. Huawei Technologies Ltd.
2012 Laboratories –Central Research Institute – Service Lab
- Developed an XGBoost-based federated learning framework with dynamic task allocation.
- Constructed an XGBoost learning model to predict the energy consumption patterns of Huawei’s 5G base stations.
Skillset
1. English skills: CET-6 626, TOEFL 110, GRE 329+3.5. Especially fluent in listening and speaking.
2. Programming Languages: Python, MATLAB, C++.
3. Coding Expertise: Data Analysis (Pandas, Scikit-learn), Deep Learning (TensorFlow), Git Version Control.
Publications
2024.01 | H. Liu, X. Zhang, H. Sun, and M. Shahidehpour, “Boosted multi-task learning for inter-district collaborative load forecasting,”IEEE Trans. Smart Grid, vol. 15, no. 1, pp. 973-986. [Link] |
2023.12 | S. Tao*, H. Liu* et al., “Collaborative retired battery sorting for efficient and profitable recycling via federated machine learning,” Nat. Commun., vol. 14, Art. No. 8032 (*Equal Contribution). [Link] |
2022.10 | H. Liu, X. Zhang, X. Shen, H. Sun, and M. Shahidehpour, “A hybrid federated learning framework with dynamic task allocation for multi-party distributed load prediction,” IEEE Trans. Smart Grid, vol. 14, no. 3, pp. 2460-2472. [Link] |
2022.08 | H. Liu, X. Zhang, X. Shen, and H. Sun, “Privacy-preserving power consumption prediction based on federated learning with cross-entity data,” in Chinese Control Decis. Conf. (CCDC), pp. 181-186. [Link] |
2022.05 | Z. Lin*, H. Liu* et al., “Tamm plasmon enabled narrowband thermal emitter for solar thermophotovoltaics,” Sol. Energy Mater. Sol. Cells, vol. 238, Art. No. 111589 (*Equal Contribution). [Link] |
2021.11 | H. Liu, L. Yang, X. Shen, Q. Guo, H. Sun, and M. Shahidehpour, “A data-driven warm start approach for convex relaxation in optimal gas flow,” IEEE Trans. Power Syst., vol. 36, no. 6, pp. 5948-5951. [Link] |
2021.07 | H. Liu et al., “Application of modified progressive hedging for stochastic unit commitment in electricity-gas coupled systems,” CSEE J. Power Energy Syst., vol. 7, no. 4, pp. 840-849. [Link] |
2020.12 | H. Liu, X. Shen, Q. Guo, and H. Sun, “A data-driven approach towards fast economic dispatch in electricity-gas coupled systems based on artificial neural network,” Appl. Energy, vol. 286, Art. No. 116480. [Link] |
2020.07 | H. Liu, X. Shen, H. Sun, and W. Zhao, “Stochastic day-ahead scheduling of electricity-gas coupled systems via progressive hedging,” in IEEE Ind. Commer. Power Syst. Asia Tech. Conf. (ICPS), pp. 64-69. [Link] |
2020.06 | W. Zhao, J. Zheng, Z. Han, and H. Liu, “Large-disturbance stability analysis method based on mixed potential function for AC/DC hybrid distribution network with PET,” IET Gener. Transm. Distrib., vol. 14, no. 18, pp. 3802-3813. [Link] |
2019.09 | X. Liu*, H. Liu*, X. Yu, L. Zhou, and J. Zhu, “Solar thermal utilizations revived by advanced solar evaporation,” Curr. Opin. Chem. Eng., vol. 25, pp. 26-34 (*Equal Contribution). [Link] |
2019.08 | Y. Wang, H. Liu, and J. Zhu, “Solar thermophotovoltaics: progress, challenges and opportunities,” APL Mater., vol. 7, no. 8, Art. No. 080906. [Link] |
2019.07 | H. Liu, X. Yu, J. Li, N, Xu, L. Zhou, and J. Zhu, “Plasmonic nanostructures for advanced interfacial solar vapor generation,” Sci. Sin.-Phys. Mech. Astron., vol. 49, Art. No. 124203. [Link] |
2019.04 | J. Liang, H. Liu, J. Yu, L. Zhou, and J. Zhu, “Plasmon enhanced solar vapor generation,” Nanophotonics, vol. 8, no. 5, pp. 771-786. [Link] |